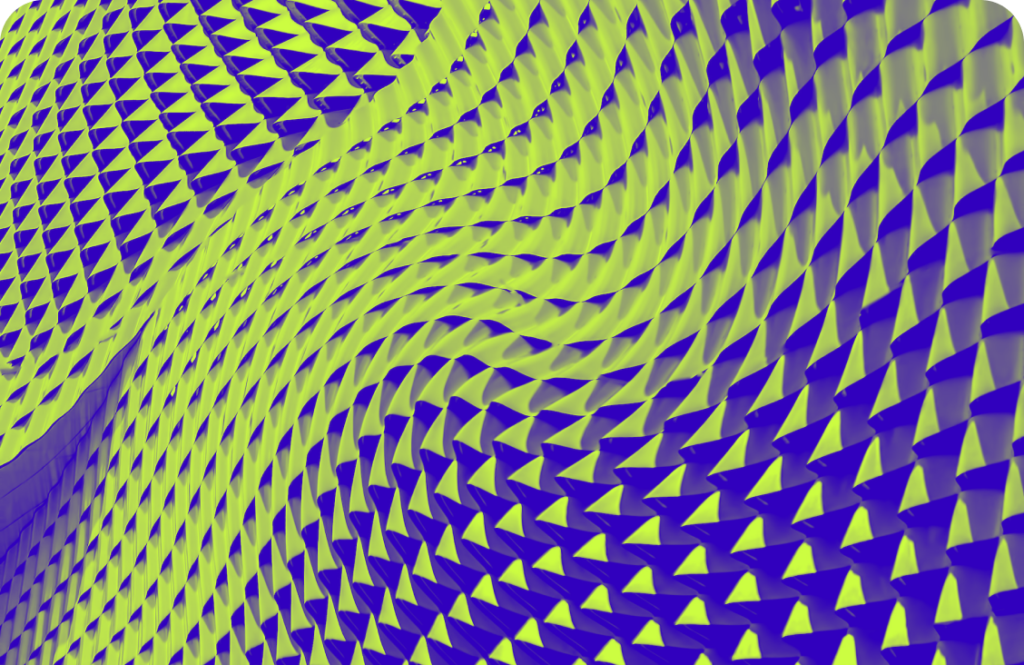
Many e-commerce companies integrate a product recommendation engine or recommender system into their website. These are designed with machine learning to suggest similar items and keep shoppers engaged. With this, there are various types of solutions used. They include collaborative filtering, content-based filtering, and hybrid filtering.
An e-commerce recommendation engine is essentially a way of automatically pointing customers to suggested products beyond what they have currently searched. It can follow them throughout various stages of the shopping process. When it comes to machine learning, this typically uses user data and engagement (clicks, search, preferences, etc) to make informed recommendations. This is usually handled via an algorithm.
Let’s discuss each type of recommendation engine along with different technologies for recommendation systems, and the best context to use them in. As with anything, there are advantages and disadvantages to the solutions offered, which will be briefly covered below. Read on to learn more.
Category management
To improve the relevancy of product recommendations, it’s advised to group products together by categories. With this, it’s worth mentioning that creating complementary clusters is more impactful than grouping similar items together. A good product recommendation strategy is built upon knowing your customers’ needs. To learn more about this topic, consult our related article on e-commerce category management.
Product recommendation engine types
As mentioned above, there are different types of product recommendation engine approaches. Regardless of which one is used, historical customer data is highly suggested to make sure your website visitors have personalized recommendations. The more data and behavior that you have, the more meaningful the suggestions will be.
The idea is to make relevant predictions on what users want outside of search-based methods. With that, the amount of information available will determine the best approach to integrate. Each type is briefly explained below.
Collaborative filtering
To enable effective collaborative filtering on an e-commerce website, it’s necessary to have pre-existing data. In fact, the more historical insight, the better. This is because collaborative filtering uses past behaviors to suggest what a similar customer will need. In general, this type uses product ratings to highlight relevant items.
Content-based filtering
Content-based filtering differs from collaborative filtering in that it’s more personalized. It also doesn’t depend on previous user behavior and makes suggestions based on preferences. These are indicated by customers when setting up an online account with your brand.
In addition, this type continues to fine-tune suggestions based on website interactions – typically handled with an algorithm. That said, it’s advised to pull in preferences from other data sources, aside from shopping behavior. For example, a profile could also include entertainment-based and news article engagement.
This requires a hybrid approach, which is further explained in the following section.
Hybrid filtering
When discussing hybrid filtering, these methods are usually a combination of both collaborative and content-based approaches. Also, other techniques may be incorporated. Hybrid filtering can use different types together or independent of one another. This type of recommendation engine is good for e-commerce websites with minimal historical data.
Of course, there are advantages and disadvantages to each type of product recommendation engine. Collaborative, content-based, and hybrid filtering are all good at suggesting items in different ways.
Advantages vs. disadvantages
Essentially, the advantages and disadvantages of different product recommendation engine types come down to how much data is available, and suggestion specificity. If there is minimal customer information, it’s best to use content-based filtering. This is because of its highly specific nature.
That said, if data is available, collaborative filtering might be better. Ideally, the products suggested should be similar enough to the customers’ interests while allowing for some flexibility. Since collaborative filtering is less based on the inclinations of a particular user, it can make suggestions outside the realm of content-based filtering.
The right approach will depend on the type of products being suggested and the availability of historical information. In general, it’s good to A/B test the best model, which will be explained following information on product recommendation engine technologies. There is no one-size-fits-all approach.
Context-aware recommendations
In addition to the types of recommendation engines listed above, it’s important to consider the context of where the customer is engaged. This means different suggestions should be used for a product detail page vs a homepage, for example. Additionally, recommendations increasingly require more personalization, through related content beyond products.
Product recommendation technologies
To support the different types of recommendation engines, there are different technologies utilized. These include session-based, reinforcement learning, multicriteria, risk-aware, mobile, and artificial intelligence (AI). They are outlined below with a brief description of each type of technology.
- Session-based
With session-based, the suggestions are given based on individual browsing behavior. This technology doesn’t use past data and is only relevant to the interactions within a single session. It’s good for sites that lack prior user knowledge.
- Reinforcement learning
As the name suggests, reinforcement learning is based on positive reinforcement to make informed decisions for users. The recommendation agent is rewarded for certain interactions and uses this insight to optimize website performance.
- Multicriteria
Using multiple criteria to make recommendations, multicriteria technology understands users across a set number of preferences. This is based on how a customer will rate various aspects of a product. Examples include sizing, color, fabric, and price.
- Risk-aware
As customers are more responsive to recommendations based on different contexts, risk-aware technology understands when it’s best to suggest products. The goal is to not turn off customers by interrupting them at inopportune moments.
- Mobile
This recommendation technology uses mobile data to provide location-based suggestions. Since mobile phones contain GPS and other functionalities not found on computers, they can recommend products differently.
- AI
The most recent type of technology, AI uses past data to automatically calibrate product recommendations. This is based on machine learning and relies less on the interactions of customers.
Looking for an AI-powered recommendation engine to create new revenue opportunities and higher your AOV? Inspire the right customers at the right time with AB Tasty. From A/B testing to product recommendations, AB Tasty is the only platform your experience optimization strategy needs.
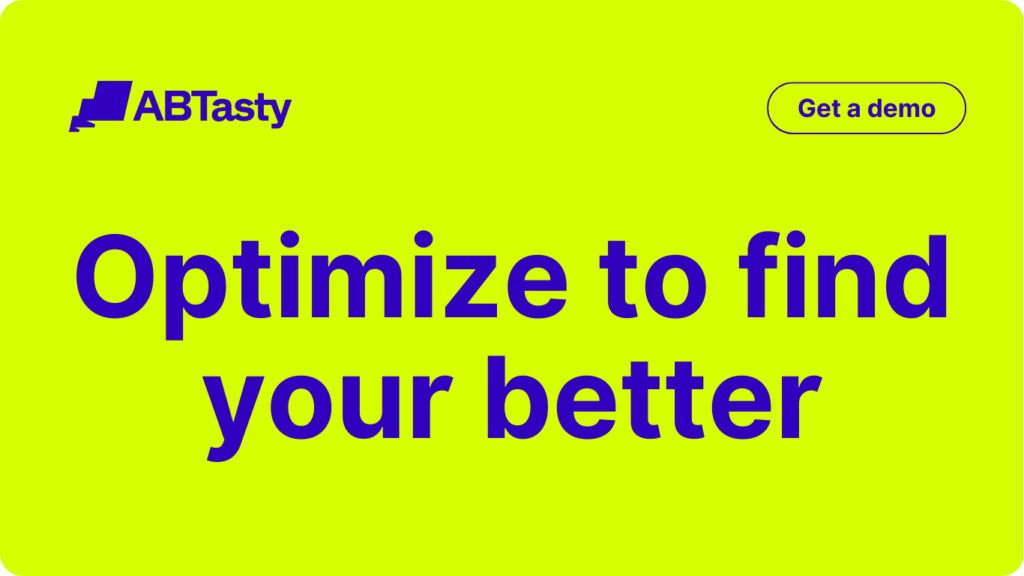
How to A/B test with product recommendation engines
When A/B testing a product recommendation engine, it’s important that conversions increase. This will indicate the right type to use. There are various ways to A/B test, including algorithm vs curated, placement, personalization, format, social proof, and dynamic vs. static.
Of course, when choosing the right method, it’s important to consider the resources available. For example, hand-curation will take more time than automatically populating recommendations with an algorithm. Similarly, researching social proof, including reviews, and testimonials takes time. The goal is to find the right A/B testing approach while optimizing operations.
Conclusion
We hope this page provides a good starting point for learning more about what a product recommendation entails. As you implement your recommendation strategy, we want you to feel confident in choosing the best approach.
Want to know more about recommendations? Keep reading our latest article here: What’s the Best E-commerce Product Recommendation Strategy?