Hello! I’m Léo, Senior Product Manager at AB Tasty. I’m in charge of AB Tasty’s JavaScript tag that is currently running on thousands of websites around the world. As you can guess, my roadmap is full of topics around data collection, privacy, and… performance.
It’s why I’m so excited to give an update on our performance, and how we’ve worked hard to be the best. We’re now providing loading times up to 4 faster than other solutions on the market.
In a world where every second counts, slow-loading pages are the fast track to lost revenue. At AB Tasty, we know that speed isn’t just about convenience; it’s about delivering the smooth, reliable experience that today’s consumers expect.
That’s why we’re thrilled to be recognized by ThirdPartyWeb.today for having one of the lowest impacts on web performance among top experimentation and personalization platforms. This acknowledgment affirms our commitment to speed, scalability, and brand satisfaction.
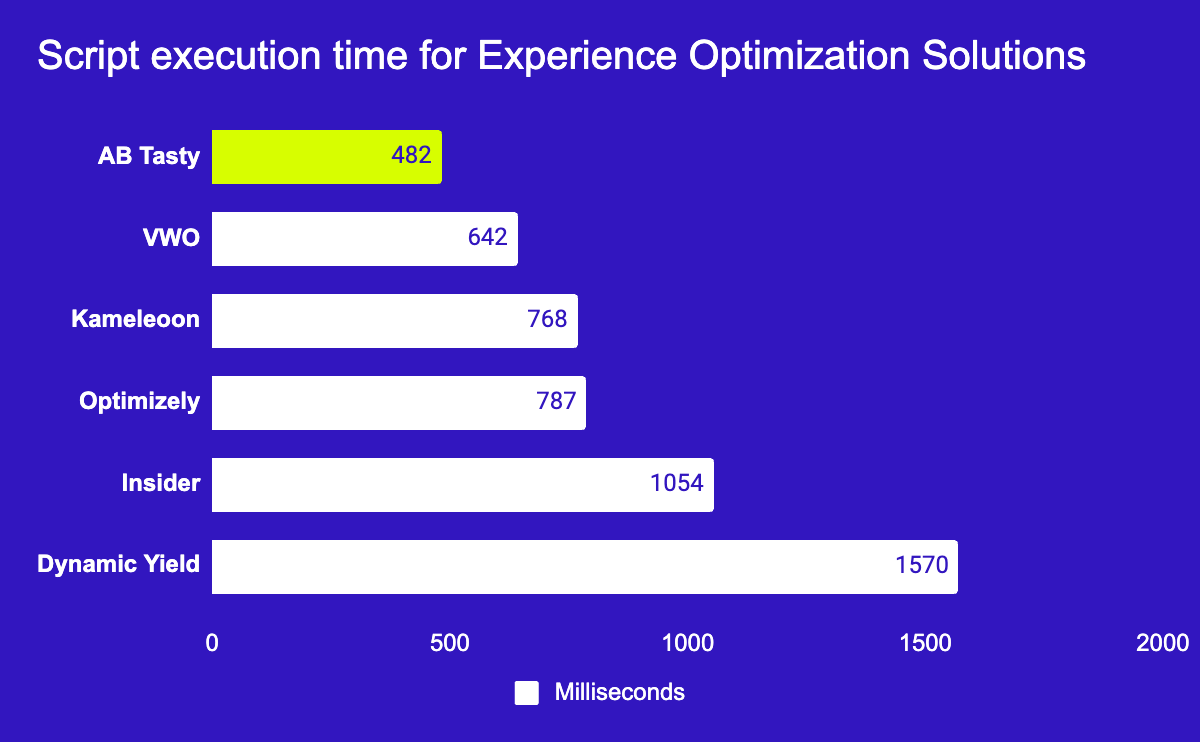
Source: www.ThirdPartyWeb.today, June 2025
But what does this actually mean for brands using AB Tasty?
Let’s dive into how prioritizing performance can improve your SERP rankings, customer experience (CX), and overall campaign effectiveness.
Why Web Performance Impacts Your Bottom Line
Imagine clicking on a page that seems to take forever to load. Chances are, you’d be out of there faster than you could say “conversion rate.” And you wouldn’t be alone: slow page load times can lead to increased bounce rates, missed opportunities, and, ultimately, frustrated visitors.
Good performance translates into smoother customer journeys, which leads to better engagement and, most importantly, higher conversion rates.
ThirdPartyWeb.today: The Performance Benchmark
ThirdPartyWeb.today is an independent performance data visualization initiative that analyzes the impact of various platforms on page speed. It ranks tools according to their performance cost, drawing data from nearly 4 million websites to create an unbiased performance benchmark. For brands aiming to deliver a seamless experience without sacrificing speed, ThirdPartyWeb.today provides a reliable guide for evaluating the performance impact of their tools.
Being recognized as one of the most performance-friendly Experience Optimization platforms by ThirdPartyWeb.today means our clients know they’re partnering with a technology designed with speed in mind.
What Makes AB Tasty the Fastest?
Our tech teams have worked tirelessly to make AB Tasty not only an intuitive experimentation and personalization platform, but one that prioritizes high performance. Here’s a quick look at the innovations that make AB Tasty so fast and reliable:
- Modular Architecture with Innovative Dynamic Importing and Smart Caching Technology
Our platform is built with a modular architecture, where only essential code is loaded for each campaign. This keeps file sizes lean, reducing load time and resource consumption. Our proprietary smart caching technology ensures that visitors only need to load the data they haven’t accessed before. By minimizing redundant data calls, we significantly reduce load times across all devices. We also provide worldwide API endpoints and have a global CDN presence with multiple Edge locations and regional Edge caches for fast response times no matter where you and your site visitors are. - Performance Center
AB Tasty’s dedicated Performance Center allows you to monitor your campaign performance in real-time. This tool gives you full transparency into what’s happening behind the scenes, so you can make adjustments as needed to keep things running smoothly. It provides recommendations to help you monitor and improve tag weight. Learn all about it here. - Single-Page Application (SPA) Compatibility
AB Tasty’s platform is SPA-compatible without requiring custom code, making it easier for developers to integrate AB Tasty into their tech stack. AB Tasty is running on a native Vanilla TypeScript framework. Our tag is compatible with modern JS frameworks, including React, Angular, Vue, Meteor or Ember. The tag is unique for all environments and doesn’t require any additional implementation. Many of our customers have left their previous provider due to challenges with SPA pages. In these tools, changes are often not “sticky” or flicker when there is a dynamic content load. SPA tests in these environments often require custom code for each test, which makes testing more complicated and less user-friendly. - Flicker-Free Experiences
AB Tasty’s tag uses a blended approach of both synchronous and asynchronous scripts to eliminate flicker, while maintaining optimised performance. Other solutions will prescribe “anti-flicker” snippets to eliminate flicker, which is not a recommended practice. It means hiding the body’s content while the tag loads, which ultimately delays the rendering of the site. This causes a worse user experience, increases your Largest Contentful Paint (LCP) metric, and may ultimately lead to increased bounce rates and decreased conversions. In contrast, AB Tasty’s synchronous tag uses 3kb of render-blocking to allow the tag to execute quickly before the page loads, as opposed to blocking the visibility of the page for the full package size.
And that translates to…
First loading time < 100ms
Caching loading time < 10ms
Execution time < 500 milliseconds
Minimal Lighthouse Core Web Vitals impact
Cheers to Our Product and Tech Teams
This wouldn’t be possible without the dedication of our Product and Tech teams (thanks team!). We’ve dared to innovate, pushing the limits of what’s possible with web performance in the experimentation and personalization space.
The Bottom Line
When brands choose AB Tasty, they’re choosing a platform that prioritizes both innovation and performance. By minimizing impact on web performance, we’re helping brands deliver faster, better experiences that delight customers and drive results.
Curious to learn more about? Contact us today to discover what else sets us apart.