Email marketing is all about maximizing your open, click and response rates while generating as many leads and sales as possible for a given email campaign.
However, in our era of over-saturated email boxes, chances are your prospects won’t actually open your emails as they receive so many.
On average, MailChimp estimates that open rates vary from 18% to 28% depending on the industry concerned. While it’s not catastrophic, it still means that 75% to 80% of your emails will remain… unopened.
Let’s be honest: there is not a single magic formula to craft the perfect email. Otherwise, it would have largely spread over the internet and become overused in a matter of weeks.
The truth is, no one can really guess the perfect email campaign for your company – it will depend on a myriad of factors that we will cover later in this article.
As a consequence, the only way to design and write the most effective emails is to A/B test them.
Not just once, but many times.
By doing so, you’ll vastly increase your chances of uncovering magic tricks that will effectively increase your open, click-through and response rates.
Using email A/B testing, you’ll also discover what actually works on your prospects and how to address them.
Without further ado, let’s begin this guide by answering one simple question:
Why does email A/B testing matter?
Despite being one of the oldest online marketing channels, email marketing remains one of the top performing solutions to reach a broad audience and convert prospects into leads or clients.
More importantly, emailing is a marketing channel that is both:
- Highly profitable
- Often affordable
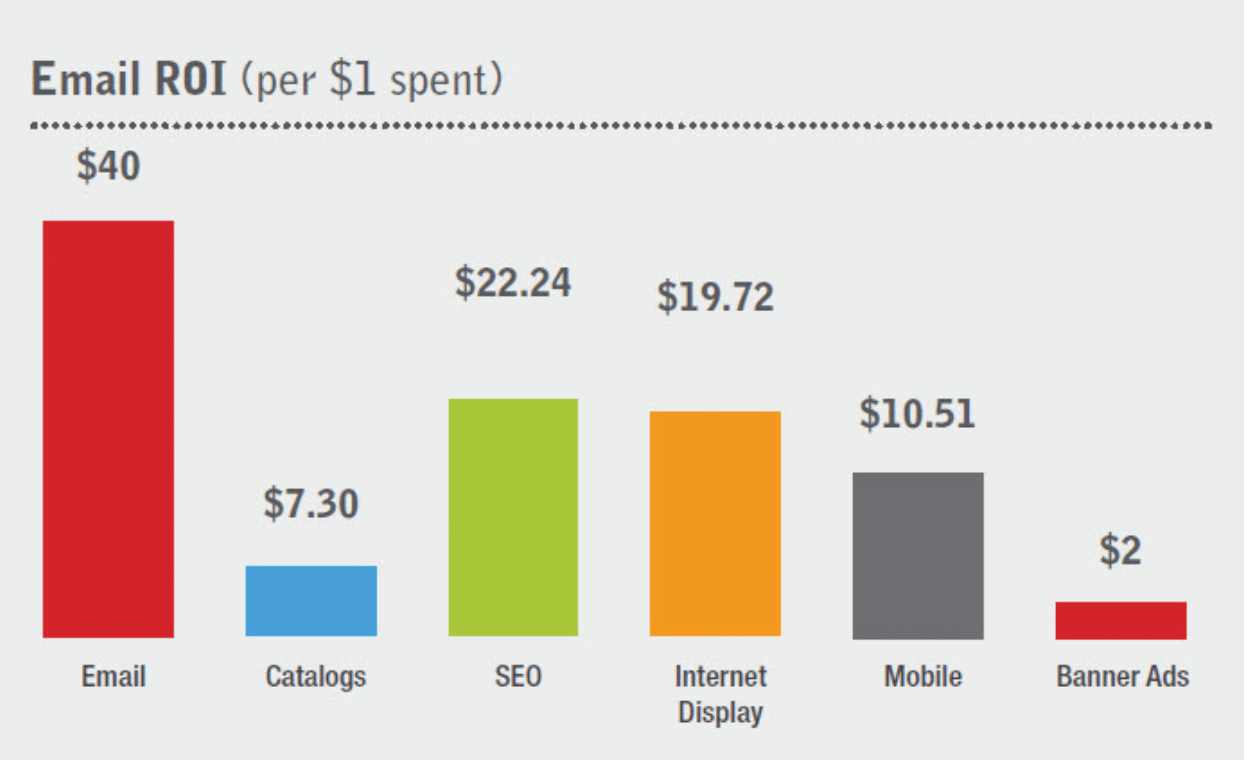
As you can see, email marketing returns an average of $40 for every dollar spent, which is a massive improvement compared to display campaigns or banner ads for instance.
Knowing that email marketing is profitable, let’s see how email A/B testing will truly help your business:
It will improve your open and click-through rates
After a few A/B tests, your company should start to identify trends and common factors that lead to higher open and click-through rates.
This means that you will get more views but also more clicks to your website or online forms, which leads us to our second point.
It will increase conversions and generate revenues
Using a marketing automation software, you will be able to analyze your funnel and traffic sources, which is crucial to identifying how many opened emails actually resulted in leads or sales.
Knowing that, you will get a precise estimation of your email marketing ROI, which is a good start to further increase conversions and revenues.
From there, it’s up to you to conduct additional tests on your email campaigns in order to generate more revenues.
You will know what works for your audience
As we said in our introduction, not all industries are identical when it comes to email statistics.
Meanwhile, your prospects most likely have special needs and questions that need to be addressed in a specific way – which most marketers won’t be able to do on the first try.
After you’ve conducted a few conclusive tests, you’ll soon discover major differentiating factors that will account for your future email marketing campaigns success.
Using A/B tests, you’ll be able to craft tailor-made emails that will fit your prospects and generate more engagement.
You will save time and money
Although email marketing isn’t the most expensive online channel, it does cost a significant amount of money to send emails to a large audience and create adapted visuals, landing pages and forms.
Using email A/B tests, you’ll save time and money by quickly identifying the recipe for success in your given industry and by implementing incremental changes that will lead to better results.
What elements should I A/B test first in my emails?
At this point, you’re probably wondering how to set up a proper email A/B test and start gaining insights on what works and what doesn’t.
In order to help you do so, we’ve prepared a list of the 8 most important elements that could lead to significant improvements for your email campaigns.
Ready?
Subject & Preheader
Subjects lines and preheaders form the only touch point before an email is opened.
Therefore, they’re highly valuable items that require extensive attention despite their size.
Remember: your headlines and preheaders will determine whether or not your emails will be opened.
On average, optimal length for email subject lines is around 60-70 characters, no more.
You could try to tweak several parameters for your subject lines, including:
- Word order (try reversing the order)
- Tone (neutral, friendly, provocative)
- Length (try shorter, try longer)
- Personalization (try including their first name)
When it comes to preheaders, they’re usually pulled from the first line of your email. But as your email marketing senses sharpen, you could try to create intentional preheaders that most emailing tools now support.
If you can create your own preheaders, try to write complementary information and add relevant words that could trigger your prospects’ curiosity.
Different days and hours
For various reasons, email campaigns don’t perform the same depending on when you send them.
For starters, you could try to send emails on different days of the week: GetResponse reports that Tuesdays get the best open rates compared to the rest of the week, although the gap is relatively small (19.9% for Tuesdays compared to 16.9 on Saturdays).
Because studies can be biased and cultural differences can change this data, it’s important that you try different days in order to find what works best for your company.
Likewise, there are studies like MailChimp’s and HubSpot’s that tend to show a particular trend for optimal sending time around 10am to 11am.
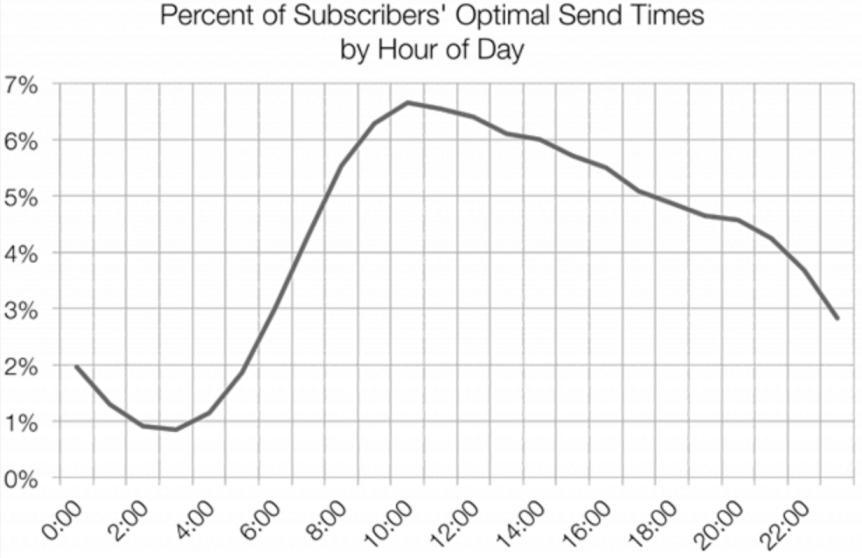
Knowing this, you could try to adjust your campaign around different hours of the day just to see if one performs better than the others.
Length
The length of your email’s body can have a significant impact on your readers’ behavior, depending on what they have been used to.
With several studies all reporting serious decreases in our attention span, it may be worth deleting one or two paragraphs just to see if your email performs better.
One general piece of advice is to be straightforward and cut out the unnecessary, overused commercial taglines.
Of course, your emails’ ideal body length will mostly depend on your prospects’ expectations and your industry’s emailing practices.
In the fashion industry, the trend is moving towards flashy, punchy visuals with minimal copy that often features a very basic call-to-action.
On the contrary, B2B emails can purposely be long and feature bullet lists as well as multiple call-to-actions.
Visuals
Since our brain just loves visuals (read full study here), adding engaging visuals to your emails can be a very powerful tool to generate more engagement from your readers.
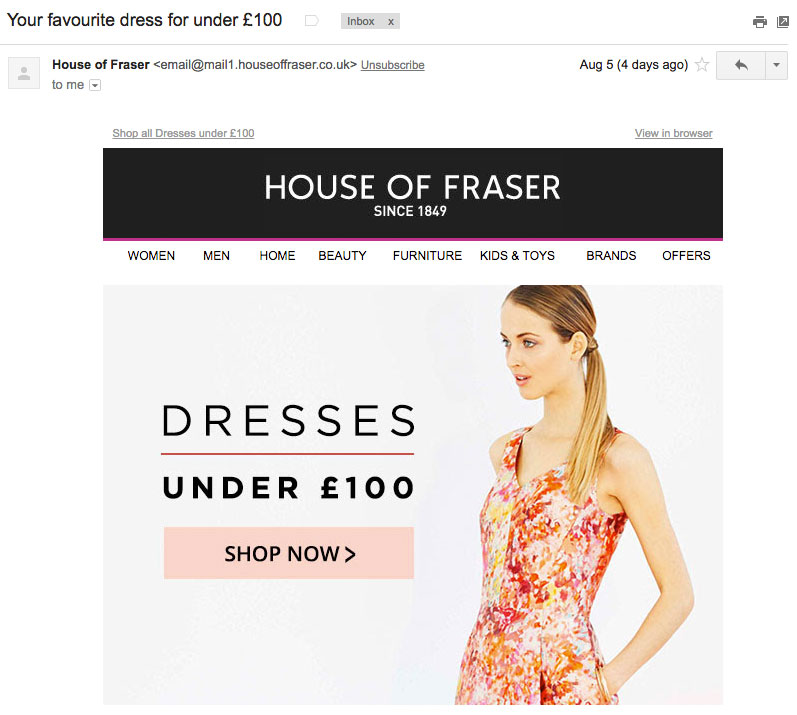
Similarly to body length, visuals won’t show the same efficiency in all industries.
In fact, adding too many visuals can distract readers from the core message which often leads to having your call-to-actions ignored.
If you want to get a clear idea on whether or not images are adapted to your email marketing efforts, just try to run a Version A with no visuals (but the same subject line, body and CTAs) versus a Version B that contains visuals: you’ll see which one performs better.
Getting more personal
Adopting a friendlier, more casual tone and copy can often transform the way your readers perceive your email activities.
Using most recent emailing tools, you can dynamically add first and last names inside your emails: this will create a sense of personalization that most people like.
The copy
While there is no secret recipe to writing perfect copy (because it depends on your objectives), try running different versions through A/B tests while only changing the copy: this could lead to tremendous changes for your conversion rate.
If you’ve formulated different hypotheses about your readers’ expectations, create two different copies based on anticipated behaviors and send them to the same mailing list to see which one outperforms the other.
Call-to-actions & buttons
Whether they’re hypertext, images or buttons, your CTAs’ design and copy can have serious consequences on your readers’ likeliness to click them.
If you want to conduct in-depth CTAs A/B testing, try to compare different colors and formats to see if one stands out from the rest.
If that doesn’t deliver statistically significant results, you could try to change your value proposition; i.e the offer behind your call-to-action.
The best practices for email A/B testing
Now that we covered the main elements that can be tested through email A/B testing, let’s have a quick look at the 4 best practices to bear in mind before running email A/B tests.
Having a goal in mind
Defining objectives prior to running any A/B tests is a massive time-saver for any marketer.
In fact, it’s highly important that we as marketers formulate hypotheses based on the data we exploit.
- You need to increase the open rate: In this case, you should mainly focus on your subject lines and preheaders: these are the two main elements that will affect this metric.
- You need to increase your click-through-rate, downloads or subscriptions: If you want to increase engagement, then test all body-related content such as the copy, the tone, the visuals and the call-to-actions as they may all trigger an increase in clicks, subscriptions or purchases.
One vs Multiple Variables Testing
When it comes to A/B testing, adding multiple variables in your tests means that you will need an ever-increasing sample size in order to get statistically relevant results.
Besides, comparing two versions with multiple variants each will make it difficult for you to get relevant results as you won’t know which element triggered an increase or a decrease for your key metric.
If you have a small sample size, our general advice is to test one variable at a time.
However, there are cases where you will want to A/B test two completely different versions of your email: you can do so easily as the “winner” could be used for future benchmarks or as a template for your next A/B tests.
Testing at the same time vs testing at different times
Although you can absolutely A/B test your emails based on sending days and hours, try to avoid sending variants at different times: you won’t know if the changes were caused by the time or the email content.
Tracking results and building on your findings
Running email A/B tests makes no sense if you don’t actively track your campaigns results afterwards.
There are 4 main metrics that should you track in order to measure success:
- Open Rate
- Click-through Rate
- Response Rate
- Subsequent Conversion Rate
For most campaigns, open rates and click-through rates will be your basic performance indicators and you should track any sensible change, be it positive or negative.
On certain campaigns (namely lead generation and ecommerce promotional offers), you’ll also want to actively track the conversion rate associated with your call-to-action.
Simply put, you should track sales or the number of forms completed on your website derived from your email analytics in order to measure your overall return on investment.
In these scenarios, you’ll be tracking real conversions instead of the number of opened emails which will provide you with much more tangible data for your marketing analysis.
Did you like this article? Feel free to share and check out our other in-depth articles on how to optimize your website, ecommerce and digital marketing.